Wildlife Co-Existence & Technology
Humans have been using their senses and intelligence to try and co-exist with animals for eons, especially when it comes to livestock agriculture. They have used other species, like dogs, to assist. But, it is difficult to have "eyes" and "ears" on all parts of a working ranch or farm. The Internet of Things and Artificial Intelligence are trillion dollar technology markets changing how people run anything from healthcare, homes, stores, factories, warehouses and cars. Often the media refers to such technology as being Smart. Smart Phones, Smart Cars, Smart Homes, etc. The agriculture industry has been moving into the space. And even wildlife and recreational management agencies are experimenting with the technology.
In Paradise Valley we are currently deploying cutting-edge (as well as established) AI technology to support the bottom-line of ag producers as well as wildlife managers and conservationists. We have found that ranchers are adopting off-the-shelf technology to support their operations, but with limited success. By bringing together new technology (e.g. AI low-power camera traps with audio and visual detection, collars, drones, and satellite imagery) and old technology (guard dogs and range riders bear proof trash cans) we intend to mitigate wildlife-livestock conflict, support co-existence, and improve the bottom line of ranchers (e.g. monitoring calving areas). Our partners in this effort include MSU-Extension, USDA, NRCS, Konexios.io, GrizzlySystems.io, Microsoft, Avnet, and the Arthur Blank Family Foundation.
This effort is one of the spin-out efforts from 2019 when the Property and Environment Research Center (PERC) collaborated with the Upper Yellowstone Watershed Group on a multi-year effort to better understand landowners’ attitudes and challenges with wildlife in Paradise Valley. A detailed report was produced and is available here. PERC has also announced an elk occupancy payment system that would encourage ranchers to allow for wildlife co-existence on their rangeland (see here for more information); coupled with AI technology that can detect wildlife and automate the claims process, we believe that this model can reach scale. Recognizing the common need between wildlife managers and livestock producers to co-exist with wildlife the Upper Yellowstone Watershed Group is now participating in a cooperative project to help ranchers solve problems like livestock depredation, forage/space depredation, trespassing/poaching, and disease transmission using next generation AI and IoT technology.
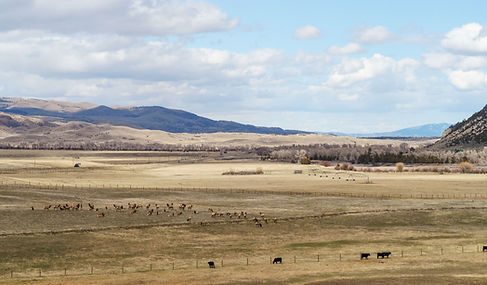%20Wes%20Overvold%20Imple.jpg)
Photography by Wes Overhold
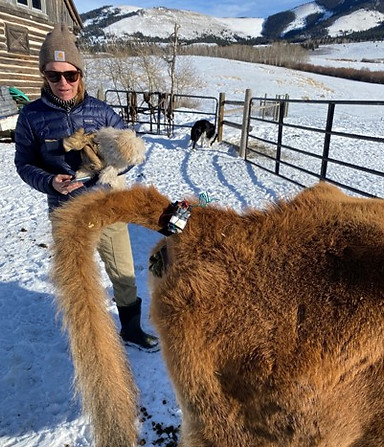
Cattle Health Monitoring Device (running on a LoRa network)
Some of the scenarios we are currently working on include:
Wildlife-Livestock Conflicts:
-
Livestock depredation (direct and indirect losses) by wild predators (e.g. wolves, grizzlies, mountain lions, and coyotes);
-
Livestock forage/space depredation by wild ungulates (e.g. elk, deer, feral swine, and wild pocket gophers, prairie dogs); and
-
Disease transmission between livestock and wildlife (e.g. elk, bison, bighorns, deer, feral swine, and mesopredators) such as brucellosis, tuberculosis, CWD, and pneumonia.
Wildlife Monitoring/Management:
-
Population monitoring
-
Movement monitoring
-
Real-time conflict mitigation
-
Species detection and gunshot detection (via audio and vision RGB and thermal AI models)
Secondary Benefits for Ranching Operations Include:
-
“Work from Anywhere” video monitoring of critical ranch operations
-
Location tracking of livestock, including but not limited to virtual fencing use cases
-
Security monitoring (e.g. poaching, trespassing)
WHY?
Private working lands (65% of Montana’s 93 million acres) are more essential than ever in stabilizing land use, fighting climate change, and providing invaluable ecosystem services. Wildlife need the connected, open landscapes that are facilitated by private working lands (Gosnell, Haggerty, & Travis, 2007). However, across much of the western US, expanding wildlife populations, development, and land conversion places increasing pressure on private working lands by concentrating livestock and wildlife on a shrinking landscape, making conflict inevitable (Mosley & Mundinger, 2018; e.g., depredation, disease transmission, resource competition, etc.). These interactions disrupt operation, cause major agricultural losses, and substantial economic damage, which place owners of working land in opposition to wildlife and wildland protection (Harris, 2020; Macon 2020). Minimizing wildlife conflicts is essential to both wildlife conservation and agriculture production.
Equally important, large herbivores and carnivores are in decline globally and these species are economically, culturally, and ecologically important to many local communities. To conserve and manage these wildlife, their populations must be closely monitored. This is challenging because these species often roam over large areas and inhabit remote, rugged landscapes. Traditionally, airplane surveys conducted at low altitude and low speeds have been the only option for monitoring large herbivores and carnivores. These surveys are simultaneously imprecise, expensive, and dangerous. Airplane crashes are the leading cause of death for wildlife biologists on the job. The advent of high-resolution satellite imagery offers the potential to survey wildlife populations at unprecedented levels of accuracy without risk to biologists and at greatly reduced cost. However, objective detection of individual species in satellite imagery poses an unwieldly analytical barrier that is inhibiting progress and undermining conservation efforts. An automated AI classification approach could prove a powerful tool for monitoring important wildlife and domestic animal populations around the world.
HOW?
Protecting livestock from predators and disease is a complex endeavor, and successful reduction of conflicts requires an analysis of the efficacy and economic efficiency of various techniques. The main goal of this project is to utilize developing technology within artificial intelligence (AI) that can be harnessed in modern, remotely deployed monitoring hardware, including trail cameras and unmanned aerial systems (drones), to provide proof-of-concept for its use in preventing wildlife/livestock conflict. We propose to evaluate if low-maintenance, scalable game cameras equipped with image recognition (smart cameras) and drones that use visual and thermal sensors can accurately classify images/thermal video and provide real-time alerts when animals that pose a risk are present on working lands. Real-time information on when and where wildlife is interacting with livestock would shift the focus of wildlife-livestock conflicts from compensation to prevention, which has major implications for production agriculture and wildlife conservation.
Future Needs: We seek to broaden the application of these techniques and to make them easy to integrate into a wide variety of management applications. Specifically, we hope to focus on:
-
Testing new smart cameras leveraging edge AI and internet of things (IoT) integrated devices on the ground and the latest in drone technology in the air (Beaver et al. 2020) to assess broad-scale adaptability and repeatability;
-
Characterizing thermal signatures for large land-dwelling mammal species and training models remotely (i.e., while deployed in the field), with particular emphasis on elk and grizzly bears; and
-
Integrating artificial intelligence and communication networks to streamline workflow for rapid, real-time multi-species detection and notification as compared to current technology;
-
Leverage AI models to do population counts of ungulates using satellite imagery.
-
Facilitating outreach to engage the broader community of landowners and managers in Montana with the study to provide peer-to-peer stakeholder knowledge exchange to successfully develop guidelines for adaptive and collaborative conflict reduction programs.
Where/When:
Montana, with focal sites in areas of concern related to grizzly bears, elk in brucellosis zones, and other select public and private lands where approval and focal overlap exist. Initial drone flights and deployment of game cameras will begin during the spring of 2021 to begin gathering machine learning data and establish protocol. Project research, data collection, and follow-up drone flights will continue in spring 2022
Expected benefits: Establishment of a reliable and cost-effective means to assess and manage wildlife populations in real time would provide a suite of practical benefits and a means of addressing further research questions for multiple species, including large carnivore-livestock conflict mitigation, feral swine control, disease surveillance, and ungulate monitoring.
Our vision is to empower a community of landowners, natural resource practitioners, and policy makers with the knowledge and tools necessary to develop a living methodology of effective and adaptive management techniques that will lead to stronger partnerships, more resilient ranches, and ultimately, better-connected landscapes.
Literature Cited:
Beaver, J.T., R.W. Baldwin, M. Messinger, C. Newbolt, S.S. Ditchkoff, and M.R. Silman. 2020. Evaluating the use of drones equipped with thermal imaging technology as an effective method for estimating wildlife. Wildlife Society Bulletin 44:434–443.
Gosnell, H. Haggerty, J. H., and Travis. W. R. 2006. Ranchland Ownership Change in the Greater Yellowstone Ecosystem, 1990–2001: Implications for Conservation. Society and Natural Resources, 19:8, 743-758. https://doi.org/10.1080/08941920600801181
Harris, R. 2020. Literature review of livestock compensation programs: considering ways to assist livestock producers with grizzly bear conservation efforts in Montana. Western Landowners Alliance. Available online https://westernlandowners.org/wp-content/uploads/2020/05/Review-of-livestock-compensation-programs-052620.pdf Accessed 8 Feb 2021.
Macon, D. 2020. Paying for the presence of predators: an evolving approach to compensating ranchers. Rangelands 42:2, 43-52. https://doi.org/10.1016/j.rala.2020.03.001
Mosley, J.C., and J.G. Mundinger. 2018. History and status of wild ungulate populations on the Northern Yellowstone Range. Rangelands 40:189-201.
Drone Technology Description:
We used drone-based thermal imaging surveys (e.g., http://tiny.cc/thermal) to document animal distributions in relation to calving pastures and estimate animal abundances. Flights for thermal imaging followed the protocol our team developed (Beaver et. al 2020). Our surveys were conducted within Tom Miner Basin, Montana (Fig. 4) via multirotor drones (Foxtech). The aircraft was controlled by a Cube Autopilot running the ArduPlane software stack (ArduPilot, http://firmware.ardupilot.org/Plane), which is common in research applications because it provides a powerful suite of capabilities in an open- source environment. We programmed flight paths using Mission Planner (Mission Planner Version 1.3, http://ardupilot.org/planner/) using the integral terrain-following feature to allow for precise, repeatable flights at a fixed altitude above-ground-level (AGL; postflight data logs show altitude on mapping legs of flight were constrained to within ±2.5 m with little to no variation in speed). The drone was hand-flown during take-off and landing using the remote-control system while all other phases of flight were under autopilot control and monitored using Mission Planner.
The aircraft was equipped with a dual-senor camera providing 640 × 480-pixel nonradiometric thermal infrared imager and 4k visible light imager (FLIR Duo Pro 640, 13-mm lens, 45° horizontal FOV, 30 Hz; FLIR Systems, Inc., OR, USA; Fig. 5). All sensors were fixed to the airframe, flight plans identical, and thermal sensor internally calibrated, ensuring repeatability among flights. The camera was mounted looking vertically downward to obtain the nadir view, and the horizontal axis of the camera was aligned perpendicular to the flight path. This sensor was self-calibrating, with a precision of 0.05° C (<50 mk).
We made 4 total flights, with morning flights conducted from 7 June 2021 through 10 June 2021. All flights were initiated within 1 hour prior to sunrise. We performed flights at 100 m AGL (±2.5 m), providing an 84-m horizontal field of view and a resolution (pixel-size) of 13-cm ground sample distance, which determines the size of the smallest object that can be resolved in imagery. The aircraft flew at a nominal ground speed of approximately 21 m/second (75 km/hr) in a Southeast–Northwest-oriented transect pattern, with 18 parallel transects spaced evenly across the study area (Fig. 6). We collected video and telemetry data continuously in flight. After flight, we trimmed telemetry logs from the autopilot to correspond with video clips, reformatted for ingestion into ArcGIS Full Motion Video (FMV; Environmental Systems Research Institute, Inc., Redlands, CA), and multiplexed the 2 in FMV to create georeferenced video. The matching then had to be edited to ensure the imagery in the drone video lined up with the land coverage in ArcGIS. Each of the videos was then annotated when there was an animal in the footage to provide exact GPS coordinates of the animals' locations.
Our drone flights summer of 2022 covered 8% of Tom Miner Basin and captured 28 elk. This provides an estimate of abundance for ~350 elk at the time of the flight (June 2021). This estimate compares well to the MT FWP aerial counts conducted in April 2021 which observed 272 elk for Rock Creek to Tom Miner Basin.